2020 to 2022 is a period in startup valuation history that deserves its own chapter, or at least its own emoji: 🦄.
The number of private companies crossing the $1-billion valuation mark exploded in 2021 and 2022, making Unicorns anything but a mythical beast.
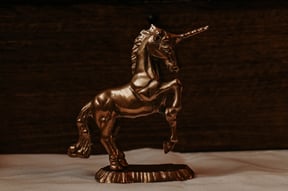
Unfortunately, the party is mostly over, and a great many of the unicorns proved to be mythical afterall (the good news: it’s still my daughter’s favorite animal).
The ever-elusive pixie dust
With changing market conditions, the CFOs of these companies discovered the real pixie dust needed to underpin a great business and valuation: a path to profitability and sound unit economics.
In this modern digital world, with great economies of scale inherent in technology businesses, why is pixie dust so hard to find?
One of the main contributing factors is surprising and vastly under-discussed: it’s the systems. With all the investment in tech a surprising number of CFOs and Controllers rely on financial and data systems born in the early ‘90s. In fact, a disproportionate amount of finance teams still leverage Online Analytical Processing cubes (OLAP cubes or Data Cubes for short), to report, measure and forecast their businesses.
Unfortunately, the OLAP cube was designed during the 90s in a very different time with totally different constraints.
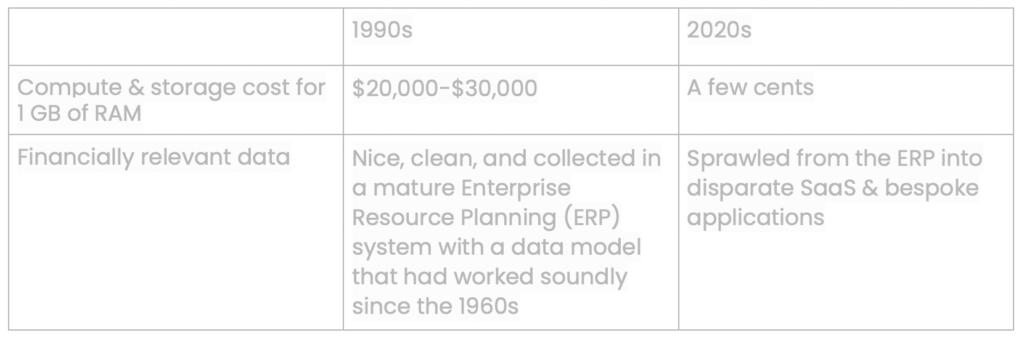
Compute has gotten many orders of magnitude cheaper, while data is spread in every nook and cranny of the business.
With such a different environment, how could the OLAP cube continue to work? Well, it really doesn’t.
The disadvantages are fairly well documented and most non-financial systems have been re-engineered for this century, but here’s a summary of OLAP Cube issues in a modern setting:
- Doesn’t play well with very granular or detailed data. This means that it may not be possible to analyze data at a very detailed level or to perform complex calculations.
- Limited data types. OLAP doesn’t play nice with text or unstructured data like JSON, which is incredibly common (which means you’re going to have to rely on your data warehouse and data engineers).
- The antithesis of real-time or near real time.
- Can only read data from the data warehouse. Complex calculations or transformations need to be done manually (eg, in Excel) or pushed back into your data warehouse tooling.
- Best for small data volumes that are typically pre-aggregated.
- Resource intensive and struggles with complexity.
TL;DR: OLAP is great for providing high level, multi-dimensional analysis on highly structured, small data sets ( but with high latency) and it’s really expensive to maintain if it gets complex. Yikes.
The symptom of all that is the amalgamation between your finance/accounting teams and the operations/product of your business is non-existent and simple things like understanding location profit or having effective controls over your accounting data will start to fail.
Unless you’re as flexible as an owl, your neck hurts anytime you need to understand and analyze your business, because the technical architecture wasn’t designed to answer the questions you’re asking about how your financials/products/etc are performing. It has considerable unnecessary operational costs. And even worse, there’s an opportunity cost to having a dated mechanism to integrate business operations and finance/accounting.
For example, a few years back I saw a scenario where disputes on subscription transactions started to spike on a certain product and it had a few hundred thousand dollar net income impact to the red. Product and analytics teams didn’t catch it because their data didn’t model explicit financial records or track “receivables”, especially obscure events like disputes. The accounting team caught it and worked with engineering to fix it and prevent 10s of millions more dollars of red ink.
How did they save it? They used their daily financial data build! Had the data been manual and relied on General Ledger data and the OLAP Cube to build, we would have caught the problem 30-40 days later at close, rather than two days later.
“If you can’t measure it, you can’t manage it”
You can’t afford not to have tooling with near real time and explicit financial records modeled for your finance & accounting teams!
But back to the original point, why is all that OLAP cube & data architecture a primary contributing factor for driving profitability? Peter Drucker said it best: If you can’t measure it, you can’t manage it.
With the economic shift, we’re starting to see the belief system shift back to a more principled approach led by wartime CFOs sitting at the head of the strategic table.
Unfortunately, while trillions of dollars in capital was chasing growth at all costs, the finance systems expertise and the systems themselves that CFOs need to measure what they need to manage have been starved for investment. The arsenal of a wartime CFO is a rusty cache designed from two eras ago, it’s time for retool (and a reorg for that matter!).
Instead of using an old design to solve modern problems, you need a modern design — intentional, precise, and effective — to solve today’s problems. You need finance systems experts and the data platforms they oversee.
I look forward to the day that modern finance systems experts and platforms are no longer Unicorns, but instead are seen as fundamental and necessary in your organization and tech stack.